This article has been reviewed according to Science X's editorial process and policies. Editors have highlighted the following attributes while ensuring the content's credibility:
fact-checked
trusted source
proofread
Self-supervised CNNs for accurate segmentation of overlapping field plants
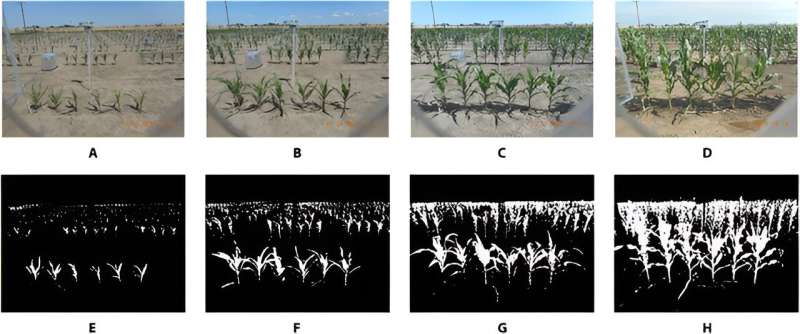
High-throughput phenotyping has significantly advanced plant data collection in agriculture. However, challenges arise when accurately segmenting overlapping plants in field images. Current methods, such as neural networks and K-means-assisted training, effectively process images with simple backgrounds but falter with complex, overlapping plant scenarios.
The pressing research need is to develop an automated machine learning technique for segmenting overlapping plants in fields that doesn't rely on labor-intensive human-labeled data, which is crucial for precise growth analysis in space-limited experimental fields.
In May 2023, Plant Phenomics published a research article titled "High-Throughput Field Plant Phenotyping: A Self-Supervised Sequential CNN Method to Segment Overlapping Plants."
In this study, a self-supervised sequential convolutional neural network (SS-CNN) specifically designed to address the challenge of separating overlapping plant images in phenotyping was proposed. The SS-CNN model uses pixel intensities from a plant's neighborhood to distinguish between foreground and background plants, a task more complex than differentiating plants from non-plant backgrounds.
The segmented images enable accurate measurement of plant heights, contributing to the construction of complete plant growth curves. These curves were then analyzed using functional principal components analysis (FPCA) to study growth dynamics and genotype impacts.
When applying the SS-CNN to the 2017 dry field data, median plant heights for 103 genotypes were computed, and individual growth curve estimations were provided. It's worth noting that, due to storm damage in August 2017, only pre-August images were utilized for growth curve fitting.
The first two functional principal components (FPCs) explained over 95% of the total variance in these growth curves. These components were instrumental in understanding different growth rates and changes over time among genotypes. Scatterplots of FPC scores offered insights into the growth patterns of specific genotype pairs, illustrating variations in overall growth rates and changes across the growing season.
In summary, this approach represents a significant advancement in phenotyping studies, allowing for automated, accurate plant trait measurements and real-time statistical analysis in the field. Future plans include integrating this pipeline with an in-field imaging robot for dynamic, on-site data processing. This method opens new possibilities for detailed, efficient analysis of plant growth and genotype effects in phenotyping studies.
More information: Xingche Guo et al, High-Throughput Field Plant Phenotyping: A Self-Supervised Sequential CNN Method to Segment Overlapping Plants, Plant Phenomics (2023). DOI: 10.34133/plantphenomics.0052
Provided by NanJing Agricultural University